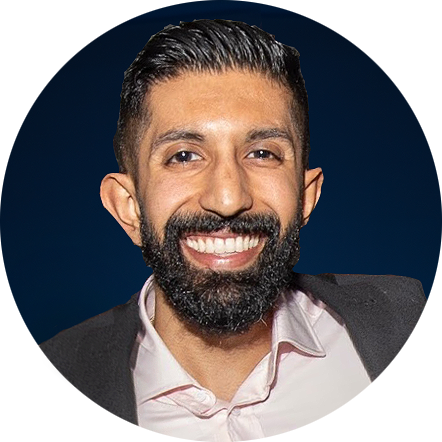
Welcome to Briefly Experimental
This edition was written by Shiva Manjunath, Senior Experimentation Strategist at Speero.
Every two weeks we'll deliver the best experimentation content and commentary, curated by a member of the Speero team. We'll break things down into the four key pillars needed for any successful experimentation program.
Edition 11, July

Strategy & Culture
🤔 How to create an experimentation strategy
This beautifully simple post from Mayank Khera, Senior Product Manager at Gojek lays out the often confused ‘strategy’ vs. ‘tactics’ definition with a great analogy. Mayank also shares three elements needed to define a good strategy, which apply to creating an experimentation strategy.
Your strategy should be;
1. Targeted. You should consider where is most valuable to experiment and set out your approach to different segments e.g paid vs. unpaid traffic, product category differences, desktop vs. mobile, etc. The experimentation strategy (in an ideal world) should be focused on ‘learning’ rather than on winning. This gives you insights that can inform future strategies. (Watch my talk about why test to learn is important, at the virtual Experiment Nation event - it's free.)
2. Consider the Future. Consider potential future roadblocks and the strategy to overcome them. E.g if company buy-in for your program is low then your strategy might include a company-wide education program.
3. Build on compounded results. As Mayank says "a bad strategy prioritizes isolated wins while a great strategy doubles down on things that can get you compounded results." Nothing is truer than this where experimentation is concerned. Setting out a strategy to prioritize iterations is crucial.
🚢 Shipping is the wrong metric focus for experimentation
Chetan Sharma wrote a fantastic article about aligning leadership and experimentation teams, in particular, the need to stop executives from micromanaging product strategy. The biggest takeaway was the necessity to shift thinking towards a metric-focused strategy, rather than a shipping strategy.
"Shipping a bunch of features doesn't mean that they've been used, that customer's experiences have improved, or that it's furthered your progress towards your mission. In contrast, experimentation (and product-led growth in general) is a great tool for pursuing a metric strategy. In essence, metric strategy says leaders should dictate outcomes, and push product strategy down to teams."
It's an astute observation. Shifting the focus towards a metric-based strategy allows experimentation to support the product team more effectively. Shipping strategies don’t allow for customer-experience-centric testing, in a robust way.
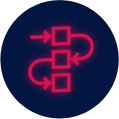
Process & Methodology
⌚ Heuristic review of WHOOP
Ever wondered how to conduct a heuristic evaluation of a website? Well, my colleague Emma Travis has just launched The Heuristic Show - a YouTube series where she'll conduct a mini-review of a different website alongside guests.
This week Emma is joined by fellow Speero colleagues, Kristel Ots and Paul Randall, as they review WHOOP.
As is usual for any Speero heuristic review, the team tracked their insights visually in a Miro board. Handy color-coding makes it easy to label insights pertaining to different heuristics around;
1. Motivation
2. Relevance
3. Clarity
4. Value
5. Friction
6. Distraction
7. Trust
Once coding is complete, the key themes or problem areas are easier to summarize by looking at the overall color mix on the board. Watch the episode as the team walks through the findings, or check out the blog post.
✋ Should you use LinkedIn polls to decide design decisions?
Recently my LinkedIn feed has been full of polls asking for votes on UI designs.
What is the purpose of this type of poll? There is an incredible amount of selection bias by ‘asking’ people on LinkedIn what they think of particular designs. You’re probably polling UX professionals and digital marketers. But who are you probably not polling? Your customer base.
So, should you use LinkedIn polls to make design decisions? Here's a handy summary of my thoughts.
What they're good for
- Encouraging good discussion
- Framing hypotheses
What they're bad for
- Everything else
It's no worse (or better) than simply applying 'best practices' to your website without testing them.
While polls can be used for feedback/discussions, the instant you use them as quantitative research, you need to stop. Immediately. Test it on your site, with real users. Research it, with actual people. Your LinkedIn audience is not your user base.

People & Skills
👀 Job opportunities
Here are a few interesting roles that have been posted in the past week.
- Experimentation Insights Director at Nike ( Oregon, US)
- Senior Experimentation Manager at Zillow (Washington, US)
- Product Experimentation Lead at Truebill (New York, US)
- Experimentation Strategist at Fanatics, Inc. (California, US)
- Insight Analyst – Experimentation at ASOS (London, UK)
- Digital Optimization Manager at Emma – The Sleep Company (Frankfurt, Germany)
- Conversion Rate Optimisation Manager at Sonova Group (Berlin, Germany)
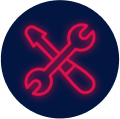
Data & Tools
Data & T
🔢 Data is more than numbers
Quantitative research often comes with the objection "that small sample sizes result in anecdotal evidence or a few people’s subjective assessments, rather than data proper."
But this objection is rooted more in a misunderstanding of why and when to use qualitative research methods. With quantitative research, we're looking to draw conclusions around a hypothesis that applies to a general population. With qualitative research, we're looking to understand a problem in detail or the "why."
Qualitative and quantitative data is like a PB&J sandwich. Peanut butter on its own? Great. Jelly on its own? Great. But when you add them together? Somehow, the flavors combine to make something even tastier.
It's the same case for quantitative and qualitative data. The combination of both research findings is greater than the sum of the individual parts. For example, your analytics data might point to users dropping out at a certain point in your checkout flow. This information alone doesn't really help you fix the problem. But with the addition of user research, you can find out why the user drops out at this point. Together you get the full picture.
This article from the Neilsen Norman Group provides a great overview of the topic. It also features a set of characteristics developed by social scientists Yvonna Lincoln and Egon Guba, to ensure a high level of rigor in your qualitative research.