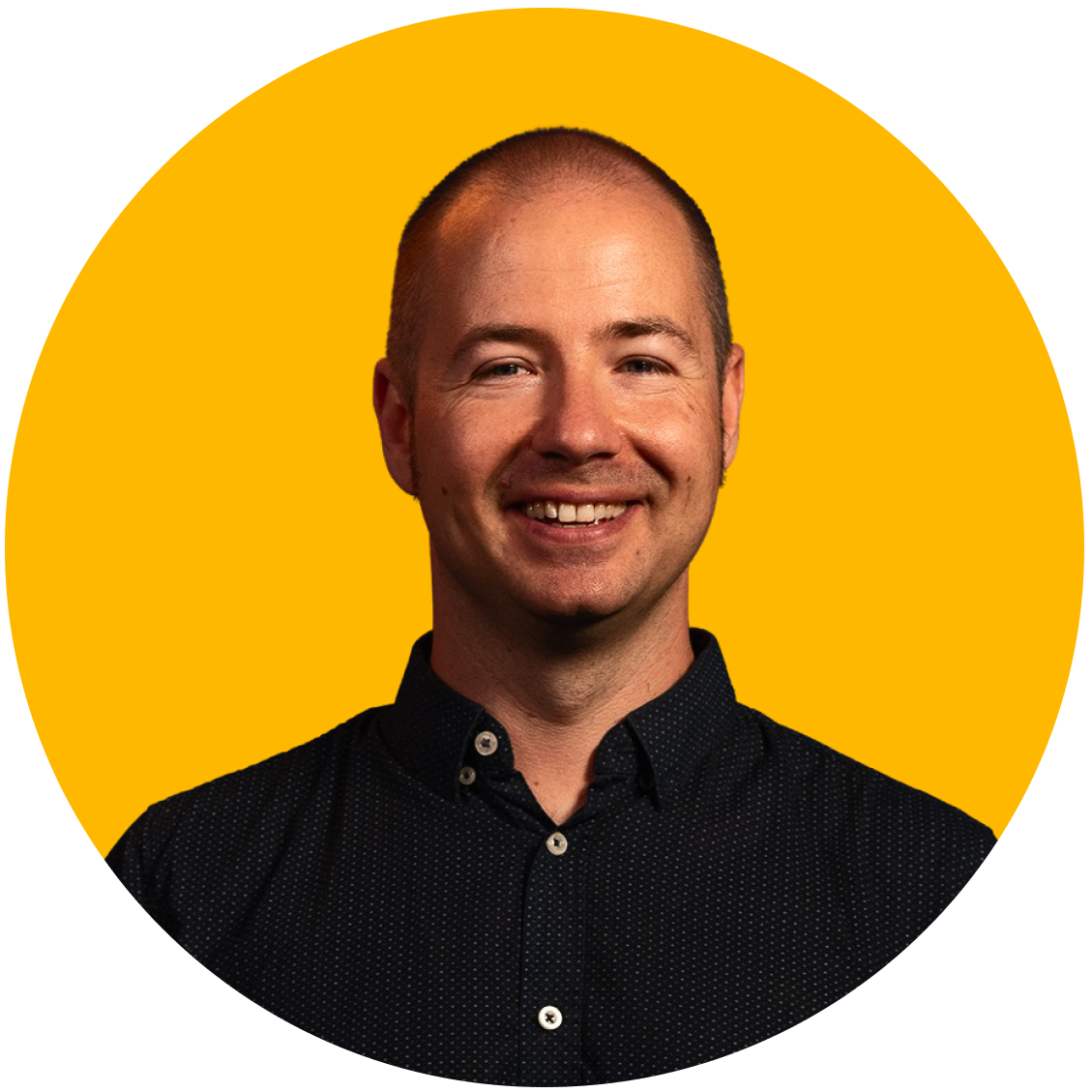
Author: Edgar Špongolts
Title: Speero Director of Product
Contact: Speak directly to author to discuss the topics
Briefly Experimental monthly aims to inform, educate and elevate the industry by sharing the latest in Experimentation learnings, and how these translate to better, faster businesses decision-making.
Edition 18, February
Key Takeways
- Establishing, nurturing, and maintaining a culture of experimentation within your organization (being data-driven) increases your performance across the board. There are almost no reasons against, but countless reasons for why organizations need to become far more data-driven in their decision-making processes. This begins by being able to describe what you do [within the organization] as a process and measure its impact. This additional transparency in itself creates tremendous value.
- Hiring the right people for the company is a huge task, but the bigger task is keeping the right people.
- Action without measurement is meaningless. Strive for a contextually fitting data-set at all times and realize that you will never have perfect data.
Headlines Of The Month
Must read article: The Experimentation Gap
Davis Treybig delivers a truly masterful piece about experimentation. The gap that he highlights between the best players out there who are capitalizing on data-driven experimentation and those who are not, is truly staggering in terms of capacity, capability as well as business growth.
QUOTE: “Cultural problems tied to experimentation can surface in a number of ways. Leaders and executives may be used to an environment where they can make gut-based decisions all the time, and struggle in cases where their gut is dissonant with the data.”
Must read article: Netflix: Lessons in Experimentation
Aakash Gupta goes over the entire history of Netflix from its inception to the present and shows how data-driven experimentation has paved the way for Netflix's astronomical growth.
QUOTE: “Netflix (NFLX) has returned 487x over the last twenty years. The Nasdaq 100 (QQQ) has returned 9.5x. The S&P500 has returned 3x”
Must read article: Experimentation and startup performance: Evidence from A/B testing
Rembrand Koning and associates deliver an extremely comprehensive analysis on the incredible impact of data-driven experimentation on startup growth and success.
QUOTE: “After a year of experimentation, the gains range from 30% to 100%. However, we find little evidence that the benefits of A/B testing vary between different kinds of startups.”

Experimentation Pillar 1 - Strategy & Culture:
THE BIG QUESTION
Does having a company strategy that is delineated into well measurable goals and milestones correlate with success metrics like revenue growth?
FOCUS
One of the main reasons why myself and the team have developed experimentation maturity [EXP-MAT] as a service is that we believe that being able to dissect and describe your processes on all levels, correlates with better results. Let's be honest; how can you truly improve a process if you don't know how it works, how to measure its effectiveness, and how it relates to other adjacent processes.
INSIGHT
After talking to a plethora of companies out there in terms of size and revenue (fortune 500 to startups) it turns out only a small (leading) minority take this stance. Most of these companies are relying on business ‘instinct’ and management opinions for critical business decisions. Just a handful of companies can actually connect the dots between their business strategy and the multitude of simultaneous processes and outputs across all departments, teams, roles, and functions. The others are still making a hell of a lot of money; they are successful and celebrated as such. So, I thought I'd see what the data says from this cross-section of companies.
As it turns out, you can of course be successful without being data-driven and make tens of millions in revenue. However, the simple, unavoidable truth is that you can remove a lot of risk from the business and make 10x more revenue IF you establish and nurture a [proper] experimentation culture.
There is a gaping performance gap, between companies that are truly data-driven and the ones that are still going with their intuition. The data is very clear; every major company displaying exponential growth is at its core leveraging business intelligence and analytics to achieve it!
CASE STUDY
- https://www.mckinsey.com/business-functions/mckinsey-analytics/our-insights/catch-them-if-you-can-how-leaders-in-data-and-analytics-have-pulled-ahead
- https://online.hbs.edu/blog/post/data-driven-decision-making

Experimentation Pillar 2 - Process & Methodology:
THE BIG QUESTION
Where does one start when it comes to becoming more data-driven and developing decision intelligence to achieve better results?
FOCUS
The beauty of being data-driven is that you can be data-driven in everything you do if this benefits the process and drives towards optimization. You can be data-driven as an employee, owner, specialist, manager, CEO, etc. The only thing that differentiates one from the other is the tools, processes, and methods that bring the best results per role or function.
As a CEO for example, you can start leveraging a wider array of research and validation methods to take the guesswork out of decision-making and crafting an execution roadmap based on the strategy that actually works and connects perfectly with measurable goals on a strategic, tactical, and operational level.
As a specialist, you can start defining and measuring the success of your work. There are plenty of frameworks available allowing you to get a running start on this no matter what you do. As you make your own work more structured and measurable and start reporting on that, others will follow. Who knows, you might even be promoted as you clearly know what you are doing.
The more people in the organization have a delineated process for what they do and a complementary set of metrics to measure the impact of their work, the easier it will become to work together. This increases efficiency and creativeness across the board as people will no longer be bogged down by needlessly complicated processes. There will be a reduction in work and responsibility overlap and who knows, maybe you guys will even create a proper RASCI matrix down the road.
INSIGHT
No matter where you are in your business, there is always something you can do to nudge the organization's culture toward more data-driven and transparent decision-making. No company was founded to be tangled up in endless red tape, internal politics, and power struggles. Being data-driven takes the ego out of decision-making and provides a refreshing touch of objectiveness and clarity into the mix.
Pro tip: look for low overhead and big impact areas first, maybe you already have some assets that are available but not used?
CASE STUDY
- When building a data-driven process make sure to make it a self-improving one as described here:
https://www.microsoft.com/en-us/research/group/experimentation-platform-exp/articles/it-takes-a-flywheel-to-fly-kickstarting-and-keeping-the-a-b-testing-momentum/ - https://en.wikipedia.org/wiki/Responsibility_assignment_matrix

Experimentation Pillar 3 - Data & Tools :
THE BIG QUESTION
What is the optimal set of measurements I should have to enable data-driven decision-making?
FOCUS
This is the toughest nut to crack as the answer depends heavily on how big you are, what is your business model and what role you are playing. The marketers would want to measure everything and the tech teams know that this can't be done. So where is the balancing point? TLDR: It's the balance between best practice and your use-case.
Start with all-in-ones testing and analytics tools. Most of the well-known tools that offer all-in-one validation and analytics tools will provide you with a good set of baseline metrics (best practices) off the bat and will allow you to even connect different data points throughout the client lifecycle (with some tinkering).
When building your experimentation system with that all-in-one tool keep in mind that you will want to ditch it in a 2-3 year period, depending on your growth curve in size and EXP-MAT (experimentation maturity). So, keep your data structure and reporting logic outside of the tool. Now, why would you ever ditch a perfectly functional toolset that I just recommended?
Because the more you work with it the more you will realize that they are catering to an average client, and there is no such thing as an “average client”. Your special requests in fine-tuning tracking, targeting, reporting will start piling up and soon it will be more effective to start building that functionality you need on your own. Especially if you own the data collection, processing and reporting logic.
INSIGHT
The key to building a good MVP for your measurement strategy is understanding the company processes and the product/service strategy. What is the range of indicators you use to establish the success rate of the company, services, or products? When thinking about the questions, try and think both short and long term. Making a sale and getting a lead is great but being able to capitalize on and retain that client is usually where the money is. So, you'd better measure the transactions as well as retention and LTV per client.
When you have the main KPI set, think about guardrail metrics; the question you should ask is “how can I predict the performance changes of my main KPIs?” and you will be on the right track. For example having a qualitative feedback system in place, like NPS scores or similar will give you an indication of pending issues with the product/service before your main KPIs start to suffer.
Bear in mind that your data will never be perfect, and that's not the goal. The goal is to have the best data possible with the resources you have and having feedback mechanisms in place to keep your data as accurate as possible. While providing enough data points for your people to measure the impact from their work.
CASE STUDY
- https://www.qualtrics.com/experience-management/customer/net-promoter-score/
- https://www.hotjar.com/google-analytics/glossary/ecommerce-tracking/
- https://towardsdatascience.com/a-comprehensive-framework-for-data-quality-management-b110a0465e83

Experimentation Pillar 4 - People and Skills:
THE BIG QUESTION
What are the key character traits and attributes that I should cultivate [or hire] to get better at being data-driven?
FOCUS
A lot of companies we are working with reach out to us to understand what kind of profiles they should be looking for to fill a CRO lead, conversion analyst, CRO pm etc roles. For the longest time, I didn't really know. I have interviewed hundreds of people and hired maybe around a hundred. Each time I think I have it figured out, the hire I was sure of underperforms or fails completely, and/or the hire I thought would be mediocre shines and grows into a leader. I think I've finally figured it out now; there are some traits that increase the success rate.
INSIGHT
Curiosity - you should always strive to understand what is the purpose of the work you are doing and how it is being measured. You can always add ‘layers’ and ask further questions to get further context” but at the core do you have a clear understanding of the macro and micro processes you're conducting?
The objective is to create maximum visibility into the context of your role, to enable you to take actions that are aligned with that context and the goals within that role. This allows for better, more insightful decision-making and more effective results.
Independence - people that just wait for the next task and stop at the first problem they encounter can't really go that far in CRO or EXP-MAT (Experimentation Maturity). The person needs to plow ahead no matter what new [data] challenge stands in their way. They'd better also know how to reframe a problem into an opportunity on the fly.
CASE STUDY
- https://www.gartner.com/smarterwithgartner/how-to-make-better-business-decisions
- https://www.forbes.com/sites/forbestechcouncil/2017/10/19/the-importance-of-hiring-curious-people/?sh=1fa50a7032c7
Join the monthly conversation with the leading minds in Experimentation by signing up to Briefly Experimental.