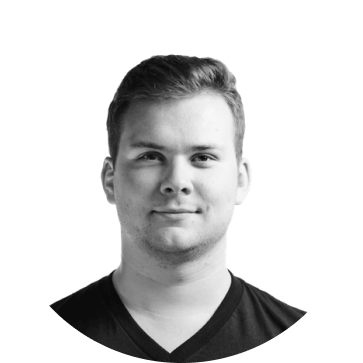
Welcome to Briefly Experimental
This edition was written by Silver Ringvee, CTO at Speero.
Every two weeks we'll deliver the best experimentation content and commentary, curated by a member of the Speero team. We'll break things down into the four key pillars needed for any successful experimentation program.
Edition 9, May

Strategy & Culture
⌛ Optimizing for long-term success instead of quick wins
Some A/B tests focus on wins that could, in the long run, hurt the business. But most aren’t measuring the impact such changes have beyond the test itself. It’s because such metrics aren’t easily measured within your testing tool or Google Analytics. To measure them you’ll need to send data from various sources into a data warehouse.
It requires some effort but measuring long-term metrics allows you to really understand the true impact on the business.
Can you answer the following questions in your business?
- Your test had a higher conversion rate for first-time purchases but what impact did it have on return business?
- The total number of new subscriptions went up. How well do they retain over 3 or 6 months compared to the control group?
- Winning variation produced a higher number of leads. How many of them turned into paying customers? What is their average lifetime value?
I'm not saying experiments that show good results on short-term metrics will have a negative impact in the long run but I also can’t say with certainty that they won't, without the data to show me.
I've also seen experiments that have a lower initial conversion rate but went on to produce lower return/cancellation rates, a higher number of repeat purchases, and overall higher LTV.
If your end goal is to improve business performance then you can’t run experiments without measuring these long-term metrics.
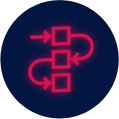
Process & Methodology
❓ Should you run that a/b test?
A/B testing isn't the only way to validate ideas.It's important to choose a method suitable to the problem at hand.My colleagues, Annika Thompson and Paul Randall will be sharing some alternatives to A/B testing for different situations, such as tree testing, copy testing, and 5-second tests, in a talk at the Experimentation Nation conference. It’s free and well worth checking out.
To illustrate this point, David Kastelman and Raghav Ramesh from DoorDash were working on a feature called SOS pricing (higher delivery fees during demand peaks). The issue with using standard A/B testing was that both sets of consumers share the same Dasher fleet — so adding a user to the treatment group also affected the experience of users in the control group. This meant they couldn’t establish independence between the two groups–known as the'Spillover effect'. Similar issues arise when running tests in social networks, or where you are looking to impact engagement or collaboration between users. Dennis Meisner, Product Growth at Facebook has written about overcoming such issues with Cluster sampling.
For DoorDash, the solution they chose was to use switchback testing.In this article, they give a detailed overview of why, in some cases, the spillover effect(which they refer to as network effect) can invalidate standard A/B tests and how their switchback test provided a route to validating their ideas.

People & Skills
🕵️ Hire and cultivate analysts not report monkeys
Producing reports and dashboards isn't what makes data valuable. It takes a damn good analyst to uncover golden nuggets and turn them into something that takes a business to a whole new level. But as an industry our hiring and training practices mean we value highly technical 'report monkeys', overcurious critical thinkers with persuasive communication skills.
Benn Stancil of Mode shares some very interesting thoughts on why and how businesses value analysts. For me, one of the main takeaways was the reminder that alongside engineers, it's the analysts and their hard work that are the backbone of a modern business, if we encourage and support them in the right ways.
👀 Job opportunities
Here are a few interesting roles that have been posted in the past week.
- Head of Experimentation at Zalando (Berlin, Germany)
- Experimentation Evangelist at M&S (London, UK)
- Experimentation Specialist at RS Components (Remote, UK)
- Conversion Rate Optimisation Manager at Boomin (Remote, UK)
- CRO Lead at Freedom Finance (Manchester, UK)
- Optimisation Manager at ASDA (Leeds, UK)
- Conversion Rate Optimizer at SwimOutlet.com (Remote, US)
- Sr. Product Manager, Experimentation Platform at Playstation (California, US)
- Experimentation Director at Comcast ( Pennsylvania, US)
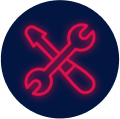
Data & Tools
Data & Tools
👇 Off-the-shelf customer data platforms have serious shortcomings
I'm a big fan of breaking data silos, consolidating data from various sources, and working towards having a single source of truth.
Recently I've seen many people thinking that a customer data platform (CDP) will solve it for them. Yes, a CDP can combine customer data from different sources and draw a unified view of a specific customer but it comes with quite a few critical limitations.
Tejas Manohar, co-founder of Hightouch and formerly an engineering manager at Segment shares five reasons why CDPs aren't ideal and why a data warehouse is a much better solution for handling your customer data.
In my opinion, a CDP is something that can be part of your tool stack, making it easier for marketers to act on customer data. But it shouldn't be the core of your customer data infrastructure. For that, you need a data warehouse.