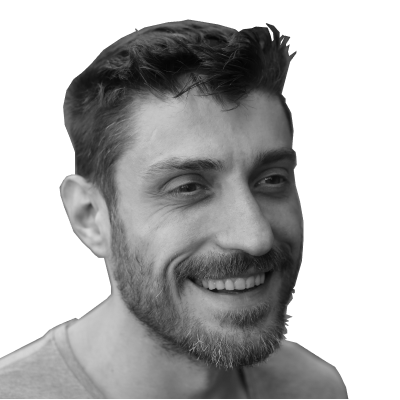
Author: Martin P.
Title: Content Marketer
EP 23: Experimentation Decision Matrix
Test, learn, win, adapt: make your clients happy.
Hi all. Martin P. here.
It’s time for another barrage of podcasts, articles, blueprints, and news from the world of experimentation.
This Week in Experimentation:
Blueprint of the week: Experimentation Decision Matrix — tag and categorize post-test results based on your hypothesis and the next action to take. Link.
Talk of the week: The Increasing Newsletter Subscriptions Test. Increasing mobile newsletter signups by giving users multiple opportunities to sign up for the newsletter by adding a simple ‘Remind Me Later’ button on a modal pop-up. Link.
Read #1: Understanding the collective impact of experiments — How Etsy uses holdout groups to measure the collective impact of all their autonomous experiments. Link.
Read #2: Mitigating the winner's curse — How to use bayesian statistics to break winner's curse, the naive overestimation of visible lifts from winning experiments. Link.
Opinion of the week: Why digital analytics and CRO/AB testing should belong in the same department. Link.
Event of the week: Accelerating innovation with A/B testing — Learn how to design, measure, and implement A/B tests you can trust with one of the biggest experimentation experts Ronny Kohavi. Link.
Blueprint of the Week: Experimentation Decision Matrix
When running experiments systematically, you need to have an action plan before you start any test.
Based on your primary success metric —and supported by the secondary ones— what's going to be your action after concluding the test?
This framework will give you a reference on how to tag and classify your results depending on the type of hypothesis (superiority vs non-inferiority) and the action to take afterward.
It will also help you enrich a narrative where “winning” and “losing” is not necessarily what’s impactful for the business.
Use Cases:
- Making a decision on whether to implement, iterate or abandon a hypothesis
- Update your experimentation roadmap based on prior results
- Classification of experiments in your Knowledge Database
Talk of the Week: The Increasing Newsletter Subscriptions Test
The Cro Show #045. Link.
This fashion e-commerce brand was looking to increase mobile newsletter signups by giving users multiple opportunities to sign up for the newsletter by adding a simple ‘Remind Me Later’ button on a modal pop-up. Do you think this increased overall signups and down-funnel metrics? The answer may surprise you. The Cro Show #045. Link.
Reads of the Week:
Read #1: Understanding the collective impact of experiments — Link.
Etsy is all about autonomous experimentation, with teams making and running their own tests to explore how users interact with new features or ideas. While this provides teams with initiative and autonomous decision-making, it also creates a complex ecosystem where tests end up running on the same pages or products.
Isolated experiments only show the marginal contributions of the associated change. For experimenters, this is enough, but a business needs more. Companies need to validate the entire group of changes that occurred.
Etsy is trying to solve this problem by using holdout groups to assess collective impact.
For the entire duration of the quarter, a small portion of our online traffic is excluded from experimentation. The excluded traffic forms a holdout group of users who are not exposed to any treatments, while they experiment on the remaining eligible traffic.
Read #2: Mitigating the winner's curse. Link.
We choose which changes to deploy based on A/B testing. Test a group of users, spot a positive lift in a metric, and qualify it as a statistically significant WIN. Then we deploy it at scale.
But if we try to track the underlying effect of that winning test, naively taking the visible lift at face value, we may overestimate the lift. A lot.
This phenomenon — often known as the winner’s curse — is a built-in limitation of our decision-making protocol. It is an artifact of how experimenters select winning treatments and it plagues how we estimate their impact, despite our best intentions.
In this article, Etsy will present techniques from Bayesian statistics that can help break this curse, by discounting reported lifts to counteract the tendency toward overestimation.
They’ll discuss the challenges and benefits of this methodology, and how it has led us to a more accurate accounting of business impact for experiments at Etsy.
Opinion of the Week:
By Johny Longden.
“It's always baffled me how many companies keep digital analytics and CRO/AB testing as completely separate teams. In many businesses, they very rarely speak to each other.
Digital analytics is the interrogation and interpretation of digital customer behavior. What reason could there be for doing that unless it was to try and improve some aspect of the digital experience? Why would you attempt any improvement to the digital experience without testing?
CRO/experimentation is about making improvements to the digital customer experience. That experience is tracked with digital analytics which is, therefore, it is one of the best sources of data to identify, quantify, prioritize and measure ideas and their outcomes.
These two things are just parts of the same endeavor, and yet they are often bizarrely considered to be relatively unrelated.
As with all the other strange quirks that happen like this, the answer lies in the lack of understanding of what experimentation is and its benefit and its incorrect application as 'CRO'.”
Link to original LinkedIn post.
Event of the Week: Accelerating Innovation with A/B Testing
Learn how to design, measure, and implement A/B tests you can trust, and live with one of the biggest experimentation experts Ronny Kohavi.
5 live sessions: Jan 30, 31, Feb 2, 6, 9 (8-10 am PST)
Lifetime access to session recordings, resources & community
The expense to your L&D budget: USD $1,600 per seat (increasing Dec 16th)
Here’s what is in store for you:
— The motivation and basics of A/B testing (e.g., causality, surprising examples, metrics, interpreting results, trust and pitfalls, Twyman’s law, A/A tests).
— Cultural challenges, humbling results (e.g., failing often, pivoting, iterating), experimentation platform, institutional memory and meta-analysis, ethics.
— Hierarchy of evidence, Expected Value of Information (EVI), complementary techniques, and risks in observational causal studies.
PS: Think someone else could use Speero’s CRO and Experimentation Blueprints™?
Share the newsletter with them.