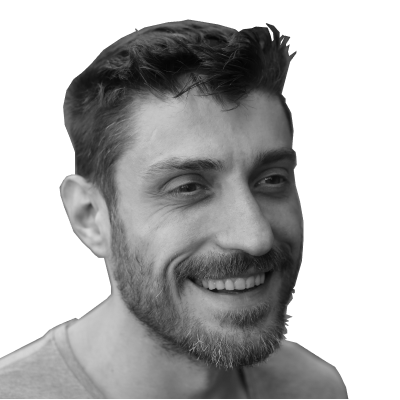
Author: Martin P.
Title: Content Marketer
The latest tools and content to help you fight the good fight. Keep on Testing!
EP 4: Experimentation Program Metrics
Yo. Martin P here.
Your eXperimental host.
Welcome to this week’s edition of the eXperimental Revolution newsletter.
Last week was about cooking the right eXperiments.
This week is about cooking eXperiments fast.
How do you measure the overall success of your eXperimentation program?
How do you oil the eXperimentation machine itself?
Framework of the Week—eXperimentation Program Metric Taxonomy

The following metrics help measure the most important factors that impact the success of your eXperimentation program and the outcomes it can generate. Grab your free template here.
eXperiment Velocity:
- Velocity: #eXperiments per year
- Law of large numbers—even if a small portion of tests win, if you run more you get more winners
- Velocity promotes/encourages/trains for action—using data to search for answers is a muscle that needs to be trained.
- Inversely related to testing complexity
- Submetrics per test category help.
eXperiment Efficiency:
- Issue rate: % experiments with production issues (ie. Dev/QA)
- can be a sign of increased complexity, so not necessarily bad
- it's ok to make mistakes, but you need to programmatically learn from these mistakes.
- Tracks issues tags from phase gates: Dev, QA, and Design.
eXperiment Quality:
- Win Rate: % of experiments with a positive effect on lead metric
- Learning or Conclusive Rate: % of experiments with impactful learnings (absolute value of an effect, so positive or negative)
- Decision rate: similar to learning rate, tests that lead to a decision (even flat tests that are run for risk mitigation lead to decisions and learnings
- Statistical significance rate: % of eXperiments that are statistically significant versus eXperiments with data trust or errors.
- eXperiment portfolio balance.
eXperiment Agility:
- # of days from insight/learning to action.
eXperiment Trust:
- Win Rate: % of eXperiments with positive impact on metrics (20-30% is quite enough).
- Fail Rate: % of eXperiments with negative impact (needs to be 30-40%)
- Error Rate: % of eXperiments that result in bad data (needs to be 10-20%)
eXperiment Efficiency:
- JDI Rate: # of changes or tweaks per month. The program can inspire many JDIs (Just Do ITs) that aren't tested.
- Decision rate: # of decisions from eXperiments or research activities per month.
- Change rate: % of website changes based on eXperiments.
- Data source rate: Ensuring ideas are based on insights from multiple data sources like user research, analytics, and heatmaps.
- Using prioritization frameworks like Speero’s PXL to systematically rate your hypothesis and focus on those most likely to succeed and increase uplift.
- Test idea execution. Using psychology principles, UX, design, creative input, and copywriting to create the best solution to the problem.
Secondary metrics:
- Number of employees trained and certified
- ROI achieved
- Implementation steps completed
- The process defined and documented
- Number of employees hired and ramped
- Number of business groups running an experiment
- Organizational maturity level advances
Talk of the Week—eXperimentation values and culture at Scale
eXperimentation values and culture at Scale—LinkedIn. Guido Jansen, host of CRO.CAFE podcast, and Alexander Ivaniuk, principal staff software engineer at LinkedIn, talk about LinkedIn’s eXperimentation, its culture, and how LinkedIn is able to eXperiment at a gigantic scale. Link.
Reads of the Week
UX of discounts. Inflation is hitting hard, and retail brands are ramping up discounts and promotions to ease its impact on customers. And their inventories, are often full of unsold items as consumers pull back on spending due to rising costs of living. But heavy discounts hurt brands. Link.
Overtracking may kill your eXperiment’s power. Pablo Estevez and Or Levkovich from Booking.com, one of the biggest eXperimentation companies (10K a year), discuss the trade-offs between detectable effects, sample size explicit, overtracking, and highlight how overtracking’s impact on false negatives and sample size can be huge. Link.
Who is trying to kill data warehouses? At first, it was ETL. Then ELT. Next came Big Data proclaiming you don’t need data warehouses. Data lakes claimed you need only them. Data mash/mesh said all you needed was high-tech data connections. And yet, all these bold swings only upgraded data warehouses. Link.
Event of the Week—How to Research Your SaaS ICP
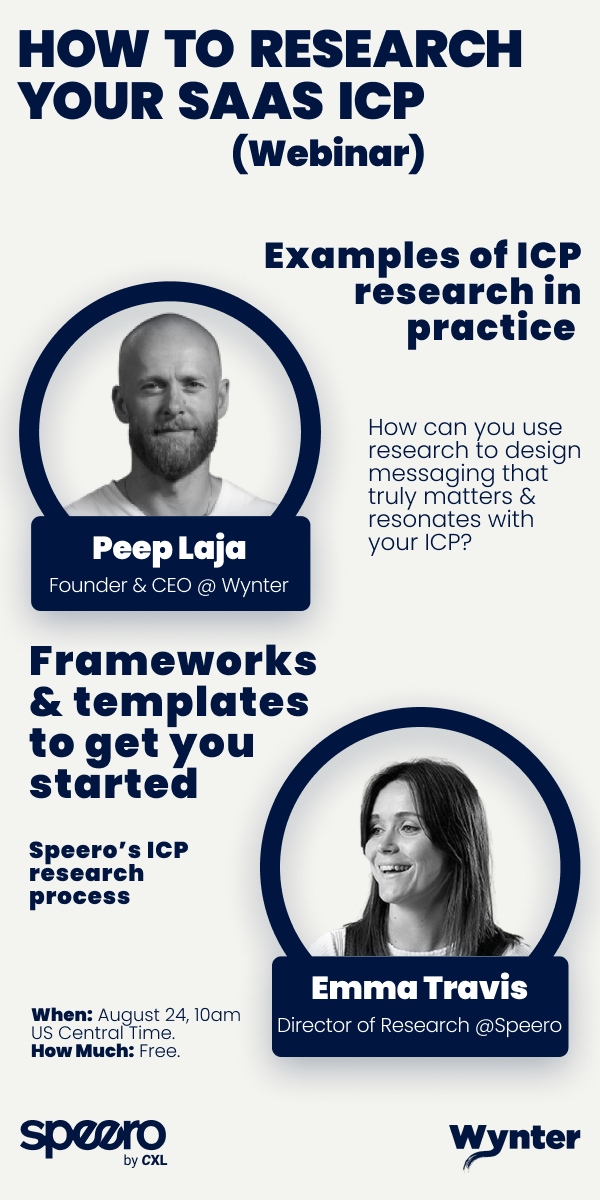
How to research your SaaS ICP. How can you utilize research to design messaging that truly matters and truly resonates with your ideal customers?
Speero’s Director of Research, Emma Travis, will show you how. How to analyze existing business data and how to turn customer insights into an actionable eXperimentation roadmap. Expect to see:
- Speero’s ideal customer profile research process
- Examples of ICP research in practice
- Frameworks & templates to help you to get started.
When: August 24, 10am US Central Time.
How Much: Free.
Register where? Here.
Martin out.
Want something for me to talk about?
You only gotta reply.
FEEDBACK & COLLABORATION
Hope you have enjoyed this month's The Experimental Revolution. If you have any feedback on the newsletter or would like to collaborate with Speero on thought-leadership content, please contact Martin P. See you next month.
Join the monthly conversation with the leading minds in Experimentation by signing up to The Experimental Revolution.