Chad Sanderson is the Head of Product for Convoy’s Data Platform team, a unique infrastructure team that takes experimentation from end-to-end. That includes everything from collecting and storing data to using it.
His team owns both the internal machine learning system and the internal experimentation system, meaning they have built their own toolset from the ground up versus relying on third-party tools.
Yet there is no one “right” way to approach A/B tools, and Chad has seen every scenario imaginable in action at his time at companies like Microsoft, Sephora, and Subway.
In the interview, Chad breaks down:
- His experience with companies that take a hybrid toolset approach
- How decision science fits into the testing framework
- The point when third party tools can inhibit the flywheel
- Why the future looks like a unified custom platform
- Why businesses should start with use cases, not tools
Do you have experience with a hybrid toolset approach?
“When I was working at Subway and Sephora, I was actually hired to bring experimentation to the company. So A/B testing was so early that they hadn't got to that point of bifurcation where the engineering team maybe realized that the third-party software wasn't satisfying their needs and then build their own thing that hadn't happened yet. But by the tail end of my time, those conversations were starting to develop,” said Chad.
Specifically, Chad encountered a hybrid approach while working at Microsoft. Microsoft had an internal tool that was used by some teams, and many other teams were using third-party tools like Adobe and Optimizely.
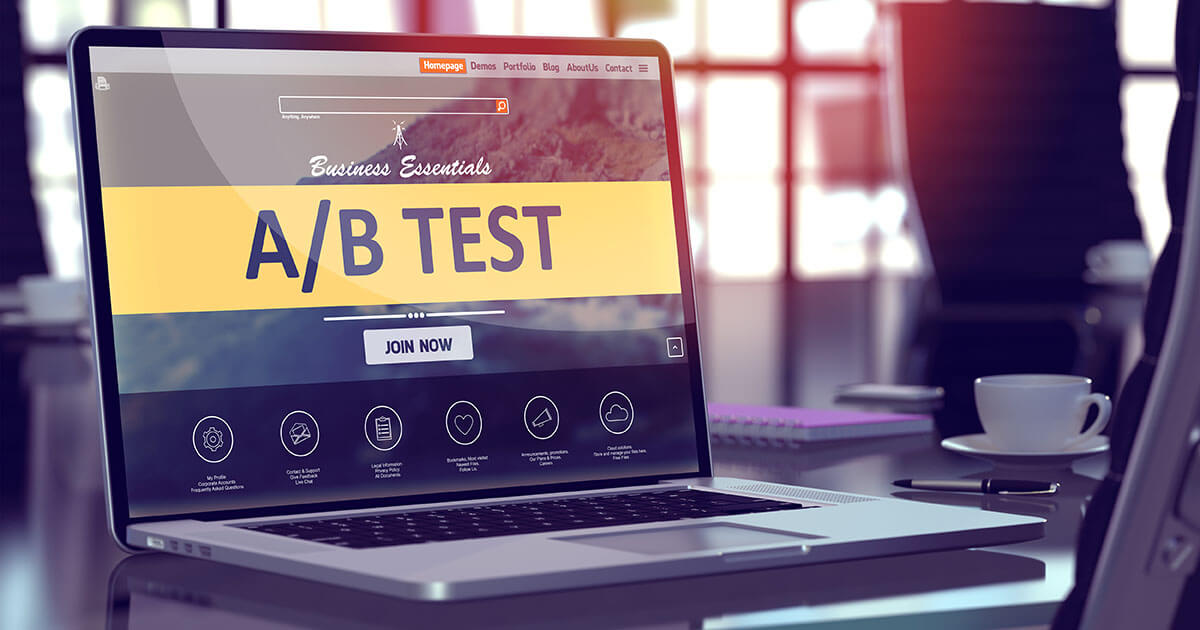
Teams seem to fall into a framework of three buckets: Marketing (third-party tools), Product (full-stack tools) and Operations (custom platforms). Would you say that’s accurate?
“Really what you're describing in the first section where you lay out the marketing use case, the product use case, and the business use case is you're talking about decision science, and different slices of decision science. You can do decision science for marketing changes, decision science for product changes, decision science for operations. To your point, you can do it for SEO. You can do it for anything. You can do it for anything that requires a change in the real world, whether it's a product feature or human beings making some alteration to an existing process,” Chad said.
All of the above scenarios have some mechanism to analyze the change, understand the change, and report the results. Chad categorizes all of those decisions under the larger umbrella term of “decision science.”
I’ve seen third-party tools speed up the flywheel. Is there a point where they can slow it down?
“The time where it's really good to use third-party software to do marketing style experiments is when you're just introducing the concept of experimentation to a new business. They really haven't heard of it before. It's not something they normally do. They don't really even think about analyzing the impact of marketing changes in that way. And so when you come in with a tool and that tool is really easy to get set up, it's one line of JavaScript, any engineer can do it, it doesn't take that long to add new metrics. Then the barrier to entry for running experiments is very low,” Chad said.
All of these factors mean that companies who are new to experimentation can get started doing tests easily and quickly, such as changing a button color, updating text, or adding new imagery. Once the team gets familiar with that workflow, it’s a comfortable baby step that can then lead them to deeper investment in experimentation culture. That said, there are downsides that can arise.
“The first one is even when it comes to the experiments that are more customer-oriented, operating at this sort of JavaScript beacon level can be damaging, and it can be negative if you build a culture based around that. One reason is because the impact of changing the user experience is not just limited to what's happening in the app. If you make it way easier to buy something or you change an algorithm, the intention is that you should be making the company money. So there's a revenue impact. And ideally there's a margin impact. And it's really hard to actually start collecting those metrics accurately if all you're using is the JavaScript beacons and sort of collecting event data from the front end,” said Chad.
The second tippling point occurs when you want to think beyond the customer, if the business has other critical elements that drive operations. This isn’t necessarily linked to business maturity, Chad explains, but rather is tied to what the business cares about.
For example, Convoy has a few important concepts outside of the customer that are critical, such at events like a shipment, an RFP, or a bid. That’s why they are consistently deploying features and process changes that are intended to benefit those particular entities. It’s why they couldn’t rely on JavaScript beacons, and made the choice to build their own tools.
Do you think that on the horizon for every company is a unified custom platform?
“I think that all companies will go to a single unified platform, but I don't think that that single unified platform is going to be internal. The reason that Microsoft and Amazon and Google all built their own system is because in order to run experiments at the data warehouse level, which is what you need in order to have access to all the different entity types, all the different metrics, you have to exist at the source data foundation. You have to be able to parse that data. You have to be able to build metrics on that. And you have to be able to understand when a particular entity has been assigned a certain experience, and do all the stitching to match all that stuff together. And then you have to be able to apply statistics on top of that data,” Chad said.
If that sounds expensive, it’s because it is. The infrastructure cost to set all of that up is enormous. And the bigger the company, the bigger the cost.
When you invest in building a platform, you can be further torn between developing it as a tool for infrastructure or a tool for marketing. Chad sees 99% of companies choose to support infrastructure, but at the bottom line the main differentiator comes down to features. If you can find a tool that allows you to invest in both directions, Chad believes that will be the unified tool of the future.
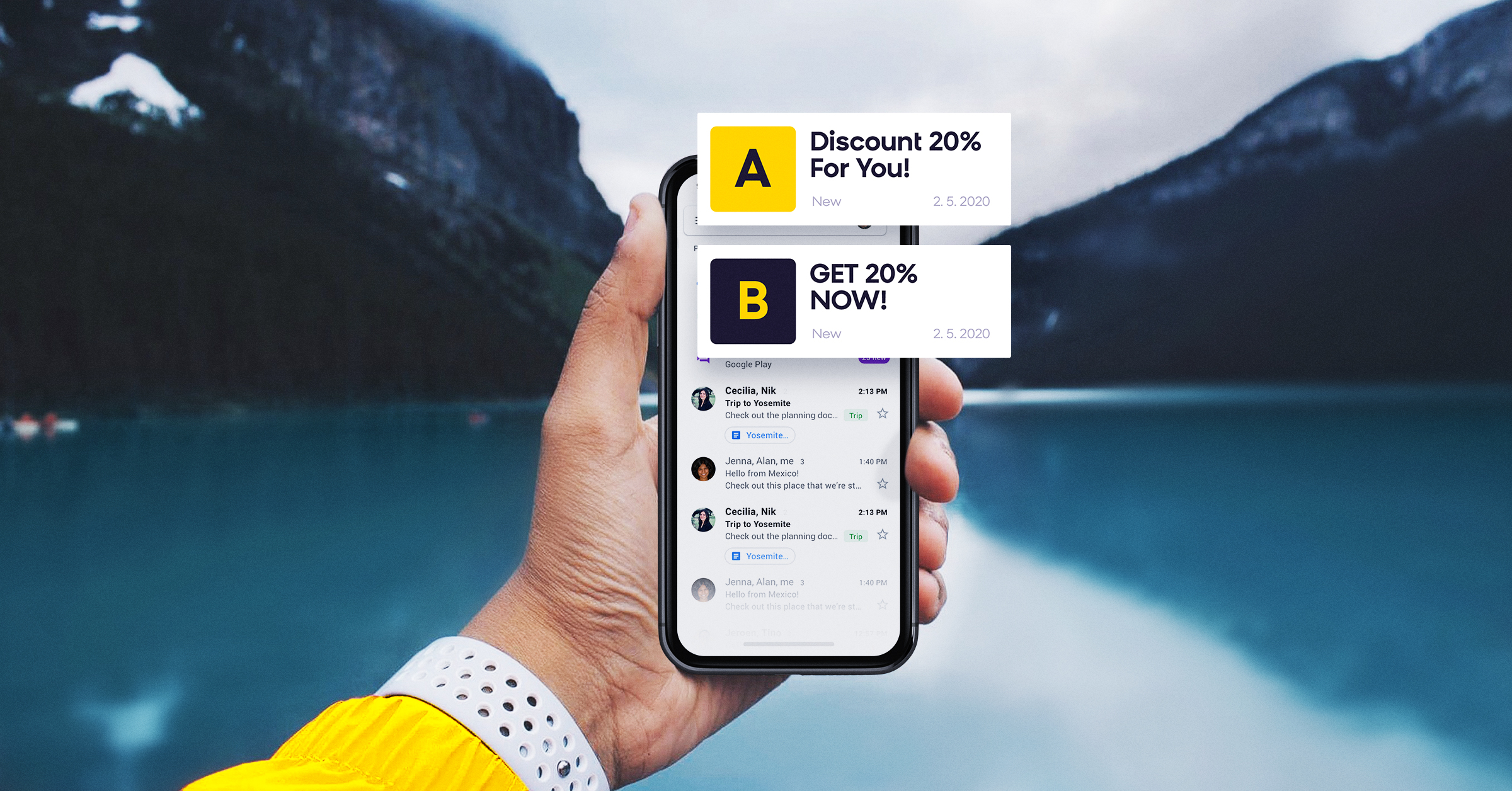
Are there any closing words you’d share on the topic of A/B tools?
“Whenever you're entering into a company that's new to experimentation, or you're thinking about what to do next, start from the most important use cases to the business. Oftentimes teams will think that there is a tool that exists, and I need to map that tool to the use cases. But instead you should say, where would experimentation have the biggest bang for its buck?” Chad said.
When you start from use cases versus tooling, you can more quickly and accurately grasp whether an existing third-party tool will work, or if you need to build a tool internally.