When it comes to enhancing your digital experience, an A/B test is one of the best ways to get decisive answers on your customer’s preferences. Whether you want to try a different checkout flow or new ad copy, it's worth considering if you should test it first.
The problem is most people have a ton of ideas but A/B testing comes at a cost–in terms of time and resources to conduct the test, as well as available traffic to test on. So you’ll need to carry out tests that have the most potential and biggest payoff.
But how can you prioritise hundreds of hypotheses to work out which you should do first (or not at all)? There are multiple prioritization frameworks out there, which you might consider using, but all come with pros and cons. To help you decide I’ve reviewed the main concepts and give you our own framework – PXL, which aims to build upon other frameworks and reduce the issues we’ve come across when using others.
What's the problem with existing prioritization frameworks?
I’ll cover three of the most popular frameworks:
· PIE framework
· ICE framework
· HotWire’s Prioritization Model
PIE framework
PIE is perhaps the most common framework, created by Chris Goward. The acronym stands for the three areas you’ll use to rate your ideas:
- Potential: How much room for improvement is there?
- Importance: What's the value of the traffic hitting the page? (e.g. audience, cost per click.)
- Ease: How difficult will it be to implement the test?

While it's the most frequently used framework, it’s open to a fair bit of interpretation which leads to it’s biggest downfall. How can you objectively determine the potential of an idea? You might think a test could completely turn your conversion rates around but by how much? More than the next test idea you have? If we could accurately estimate potential we wouldn’t need prioritization frameworks.
Similarly, how ‘easy’ an A/B test is to implement could give you a range of answers depending on who you ask. For me, there's just too much guesswork involved in this scoring system to determine the real value of a test.
ICE Score
Another popular framework is the ICE Score which was created by Sean Ellis. This framework also splits into three separate variables on which you will score an idea:
- Impact: What's the potential impact if the test goes to plan?
- Confidence: How confident are you that your idea will work?
- Ease: How easy is the test to implement?
Some useful ideas to consider, but if you're already confident that an idea would be successful why would you bother testing it?
Again, there's just too much open for interpretation and not enough objectivity. The criteria aren’t specific enough to create consistency in your ratings. Not to mention if you've thought of an A/B test idea you're keen to implement, it's far too easy to tweak the score around your personal bias and gut instincts.
Another Ice Score
Same name, slightly different concept. This ICE score also consists of three core rating factors (are you sensing a theme?)
- Impact: What is the potential benefit to the company?
- Cost: How much will the test cost to implement?
- Effort:What resources and time are needed for this test?

With this framework, we have slightly more specific criteria alongside a binary scoring option of either 1 or 2 - depending on whether the answer is "high" or "low". The small scale helps you avoid the error of central tendency (where we tend to cluster answers around a mean, mode, or median number.)
This scale also helps us be more accurate too. As Jared Spool said, “anytime you’re enlarging the scale to see higher-resolution data it’s probably a flag that the data means nothing.”
While the scale is a definite improvement, it's still not perfect. The limited prioritization factors mean you could end up having ideas that all score the same. And if that's the case, where do you go from there?
HotWire’s Prioritization Model
Pauline Marol and Josephine Foucher from Hotwire shared their prioritization model at a previous CXL Live event:
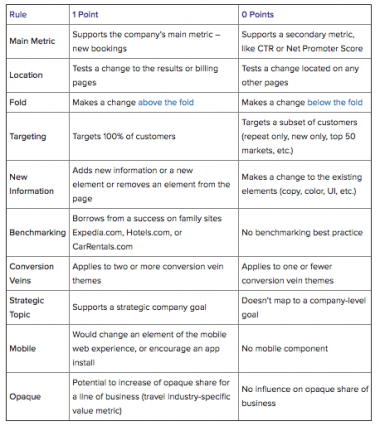
Also based on a binary model, each component scores either 1 or 0 points. From where you're making the change to the strategy behind it, this model considers a lot.
The level of specification combined with the binary approach helps eliminate subjectivity. As there are so many factors, you'll be able to use this approach to prioritize a large number of different test ideas.
Inspired by this level of detail and specification, we set about creating our own prioritization model and after trying out different approaches with clients we refined our framework.
Introducing the PXL Framework
As we wanted a framework that delivered maximum objectivity, we created our own prioritization framework. Just click the link to get your own customizable spreadsheet.
Taking on board what was lacking in existing frameworks and building upon those with potential, here's what we developed:

With objectivity at its core, this model requires data to impact the scoring. It breaks down what it means to be 'easily implemented', and instead of taking a shot in the dark regarding the potential impact of your idea, this model quantifies the potential.
The PXL prioritisation framework asks you:
- Is the change you're implementing above the fold? Changes above the fold will generally deliver a bigger impact, as they'll be more noticeable.
- Will the idea run on a high traffic page? If it's a high traffic page, winning changes have the potential to make a bigger impact on your bottom line.
- Can people notice the change in less than 5 seconds? Ask your colleagues if they can notice any difference between your intended control and variant–if it takes longer than 5 seconds it's likely to have less of an impact and if it’s not noticed let alone changing user behaviour it’ll likely end up as an inconclusive test.
- Does it add or deduct anything from the page? Changes like adding useful checkout information or removing unnecessary steps will naturally have a bigger impact.
Ideas that are derived from opinions have less chance of success - so it's well worth doing the research. That’s why our framework adds weight to ideas derived from data.
- Did you discover the issue through user testing?
- Did you discover the issue through qualitative feedback, like a survey?
- Is your hypothesis supported by heatmaps and mouse tracking?
- Are you addressing findings from digital analytics?
As these are all backed by data, you won't waste any time discussing ideas that are unfounded or based purely on opinion.
We also put constraints on the ‘ease of implementation’ by setting brackets for different estimates. You’ll want to have your test developer be part of the discussion. Over time people become better at estimation so you’ll probably find estimates become more accurate the more tests you run.
How the grading system works
While our system is a binary system, we also wanted to weigh different criteria in order of their importance. Some variables are likely to have an impact or be successful, so we give a higher score for these.
For example, the noticeability of the change will be scored either as 2 or 0 (as opposed to 1 or 0 for less impactful criteria).
Design the PXL framework around you
All businesses are different. What might have the potential to be successful for one company could be completely meaningless for another.
That's why we created this model with the flexibility for you to adjust it to your specific needs. For instance, your marketing might be driven primarily by SEO. With our framework, you could add criteria that rates the impact your idea might have on your SEO ranking - which would, in turn, affect the ranking of some copy test ideas.
Start testing better ideas today
If you want to run a successful A/B testing program it's essential to prioritize your ideas in a way that's objective and based on facts, not opinions. You can grab your own copy of our framework and save a copy to customize it. It's completely free and will save your team countless debates about which A/B test to carry out first.