Most of your tests will be inconclusive. There. I’ve said it. Even if you get your data right. Even if you take MDE into consideration. Even if you know all the basics and best practices about A/B-testing.
According to Experiment Engine’s data, anywhere from 50% to 80% of test results are inconclusive, depending on the vertical and stage of the testing program. VWO and Convert.com have produced estimates that have concluded only about 1 in 7 A/B tests is a winning test.
This image illustrates the hypothetical distribution of return on investment for ideas generated by a company (Online Experimentation: Benefits, Operational and Methodological Challenges, and Scaling Guide, Iavor Bojinov and Somit Gupta, 2022)
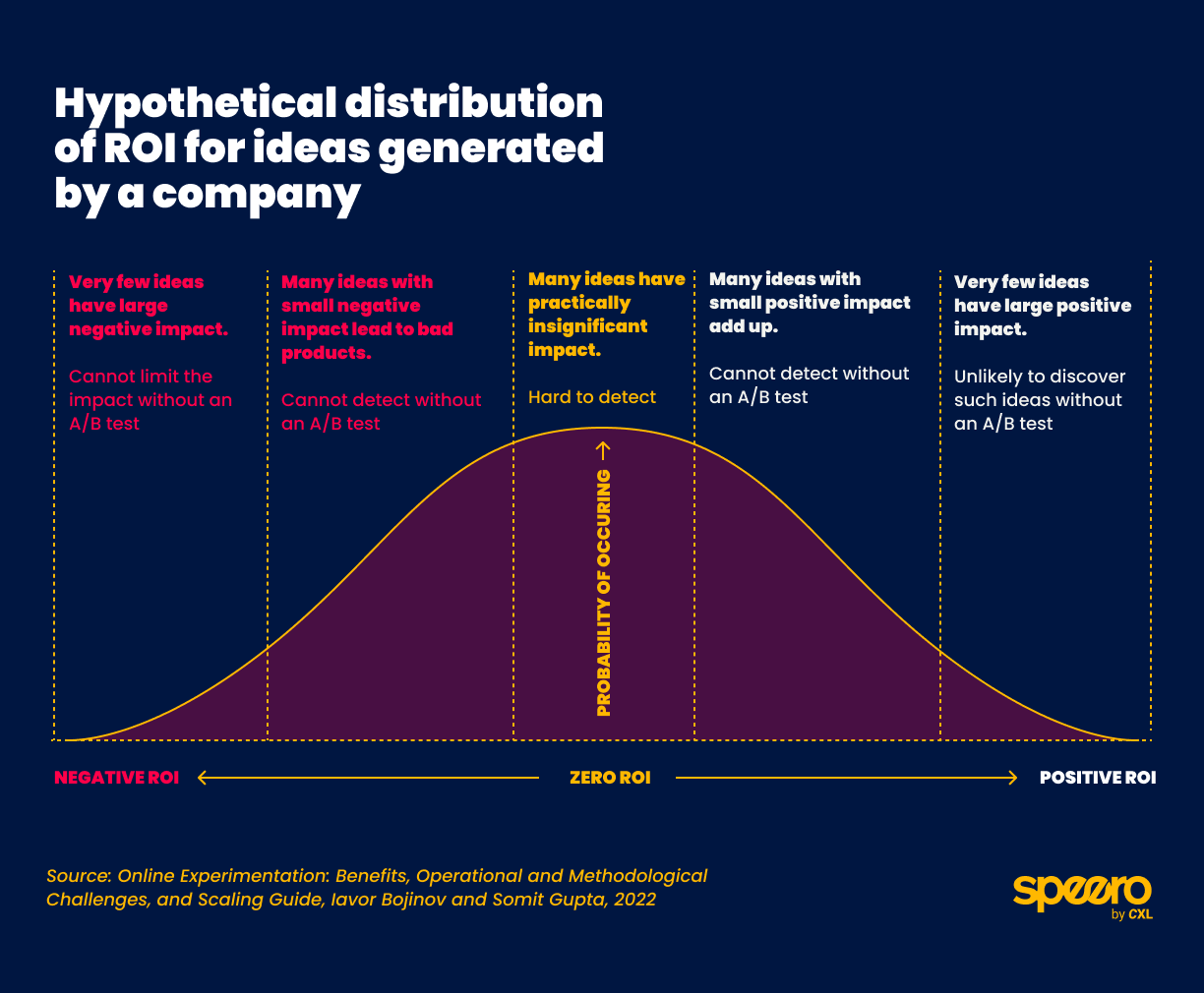
What to do with inconclusive A/B-test results?
Since inconclusive results appear to be the norm rather than the exception, what do you do when you get them?
Implement, when testing for non-inferiority
Are you testing to see if the change doesn’t have a negative impact? Then you are looking for non-inferiority. When we usually experiment, we are looking for superior results—winning variants.
But this doesn’t always need to be the case. If the purpose of your test is to learn if the change has no negative impact, with a suitable hypothesis, then you can still implement it if that is the way you want to go (with the website and/or app).
What you can look into:
- Look into your secondary, guardrail metrics. Do they tell you anything? Ideally, you’ve set these metrics before. Most eCommerce websites aim for transactions (conversions), and a secondary metric is usually add-to-carts or checkout views. A Goal Tree Map can help you explore your secondary metrics (link to another post). Also watch out for ‘data torture’, managers and stakeholders are sometimes asking questions about a lot of metrics just to find an answer. The thing is: if you dig deep enough, you will always find something. It’s a distraction, just to soothe our minds into believing we actually found something.
- Segmenting on mobile vs. desktop or return and new visitors. It’s best if you think this through before and while creating the hypothesis and test. Not as a necessity to find a ‘winner’.
- Is there another test you ran simultaneously (link to another post) that might have affected this result? You might want to filter (read: segment) that data out.
- Sometimes there is qualitative data to collect to support your quantitative data: like a questionnaire in the variant, additional visitor recordings, or heatmaps.
For next time, there might be things you can improve or check on your process:
- Are you collecting enough data? Did your test run for a representative sample?
- Did you have a strong hypothesis? Check out this Hypothesis Kit.
- Do you get a lot of inconclusive results? Check if the research and data fueling the test hypothesis are correct. Sometimes insights don’t reflect real behavior or we see things in data that we want to see.
- You could do some pre-test research to validate the change before the A/B test (tree testing, user testing, copy testing, etc)
- Did the test trigger the correct users? So, only show the tests for visitors that have scrolled 50% to the bottom of the page, or only show the test for logged in users if your test is about that.
- Small websites, with a small number of conversions, usually cannot make small changes significant. You can check your data with a pre-analysis calculator and look at the MDE. Usually, an MDE should be between 1% and 10%. With 1% - 2%, you could change the text on the page or do some small UX changes, but with an MDE of 5-8%, you’ll need to look into bigger changes to make something significant, and maybe combine multiple changes with the same hypothesis to get a result.
When do you iterate on a test?
Sometimes a change is not bold enough, and you might want to iterate or head in a completely different direction. Your prioritization should help you out with this.
If you have a new test idea from an inconclusive test, you can use a prioritization model like PXL. A high enough outcome and the test ends up on top of your to-do list. You might also want to ‘park’ ideas for a while, explore another direction, and come back to them later.
“If you find any interesting results or info for qualitative data, it could be used for the iteration. I'd say that it's worth iterating if you're sure there's a customer issue for example and the solution that would fix it is not clear. Or if you're testing for some margins/revenue metrics and looking for a "sweetspot". Annika Thompson, Account Director at Speero.
The philosophy of Accepting Inconclusive Results (Change is Overestimated)
Let me tell you something personal: I thought that a lot of my ideas, changes, or tests would have significant impacts. After running 500+ experiments I found that the reality is far from that. Most executions create only a small ripple in the behavior of your visitors, an effect that cannot be measured.
This taught me about how we generally overestimate the effect of our ideas, opinions, and changes. Most just don’t matter that much. This reminds me of two biases as well:
- Illusory superiority (a person overestimates their own qualities and abilities),
- IKEA-effect (the more time you spent on something, the more you value it, which automatically happens if you think up the idea).
Many decisions (in business or life) do not have the great effects that we hope them to have. Only around 1 in a few decisions will really blow your mind and have spectacular results.
If you simply implemented changes you’d have no idea if the change was positive, negative, or flat. You would not know if you were heading into the right direction. At least, experimenting makes ideas measurable.