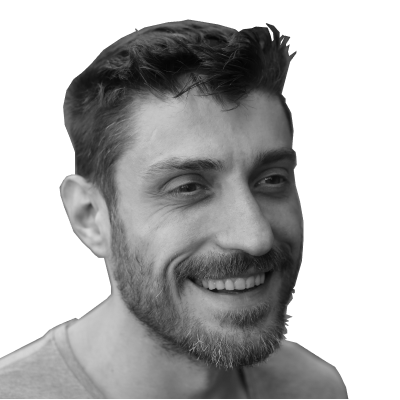
Author: Martin P.
Title: Content Marketer
EP 13: MAB Vs AB
Revolutionizing experimentation industry. One test at a time.
Martin here.
What’s up?
Welcome to this week’s edition of top info in experimentation industry.
Here is This Week in Experimentation:
— Is 95% statistical significance enough? Link.
— Attention in early design has a big impact on costs and quality down the road. Link
— Most of your tests will be inconclusive. And that’s ok. Link
— Running multiple tests at the same time is the least of all evils. Link
— Create a data-driven experimentation program with Speero’s research playbook. Link.
Blueprint of the Week: MAB vs A/B Tests
This framework is a guiding tool on when to run a multi-armed bandit vs. running a true A/B test.
A/B testing allows for a more statistically controlled learning environment, while MAB is more focused on generating a win as quickly as possible (at the sacrifice of understanding 'why')
Generally, this comes up when A/B testing may not have enough time to run (or traffic volume).
Or, there is a seasonal campaign that is a one-off (with the above parameters)—so use this framework to decide when to MAB vs. run an A/B/n test.
LInk to the blueprint.
Talk of the Week: Is 95% Enough for a Winner?
Some experimentation questions are easily answered; others not so quickly.
In many cases, there is a gray area rather than a clear guidebook.
On “It Depends,” Speero’s Paul Randall and Shiva Manjunath tacked one such important question: "Should you always go with 90 or 95% statistical significance to declare a winner?"
As you may have guessed, there is no right or wrong answer. The answer lies somewhere on a spectrum and depends on your processes and business goals.
Link.
Reads of the Week:
1-10-100 rule by George Labovitz and YuSang Chang: it costs exponentially more money to identify and correct data entry errors the longer it takes to find them.
1$ is the price to verify data as it enters the system. The least costly way to ensure you got clean and accurate data.
10$ is the price to clean the data after fact since now you have to spend a lot more time and resources. You gotta setup a team to correct errors and validate data.
100$ is the price of doing nothing. Bad data can flow between sources, making you bleed time and resources. This is failure cost.
The same is true when making decisions. Link.
The philosophy of inconclusive A/B test results: Most of your tests will be inconclusive.
Even if you get your data right.
Even if you take MDE into consideration.
Even if you know all the basics and best practices about A/B-testing.
But this isn’t a bad thing. Link.
Can you run multiple A/B tests at the same time? Yes, and its the least of all evils.
If you try to isolate tests, you experiment with fewer changes at the same time.
So you reduce testing velocity (reducing the program’s success).
If you isolate traffic, you reduce your statistical power.
So you end up with longer tests (reducing the program’s success)
There’s a better way. Link.