If you want to improve your customer experience for users, you need to consider the whole (rarely linear) end-to-end journey, for the common tasks they complete, both online and offline.

But companies are set up to operate around touchpoints, not usually journeys. Different touchpoints are “owned” in silos across a range of business functions. Which leads to fragmented experiences that don’t satisfy your customers.
And to make getting this view of the user journey even harder, not only has the number of touchpoints a customer has with businesses grown over the years, but 60% of online adults in the US use at least two different devices every day. So getting the data that shows what individual users are doing throughout your journey is no easy feat.
If you can overcome the obstacles to get data-backed customer journeys, it will pay off. Those who do so achieve 91% greater year-on-year customer retention compared to businesses that don’t, an important factor in The Retention Economy.
So how can you get this hard-earned view of your customer’s experience? It all starts with data and how it’s managed. Enter, the Customer Data Platform vs. Data Warehouse debate.
Now, no matter which you choose, this isn’t a small undertaking. We’ve previously written about how you can pitch a data strategy to the C-suite which guides you through getting buy-in for the work, and how to decide what data/tracking is needed for experimentation. A similar process should be followed across the different customer journeys your customers have over their lifetime with your business, to establish the data/sources/tracking/metrics/ you need.
Given the work involved here, it’s best to choose an option that meets your requirements today and in the foreseeable future. This will save you a lot of time and money by only doing it once. So, to help I’ve presented two options with the pros and cons of each option.
But first, what should you be looking for when considering these options?
1. Joined-up data from various sources/touchpoints
Most likely, your business is using dozens of tools that all collect data about your customers. Each tool has its own terminology, ways of calculating certain metrics, and policies for what data different teams can access. It’s messy, duplicated, ununified. These“data silos” lead to different teams reporting on similar, but different information, often leading to arguments around the data integrity.
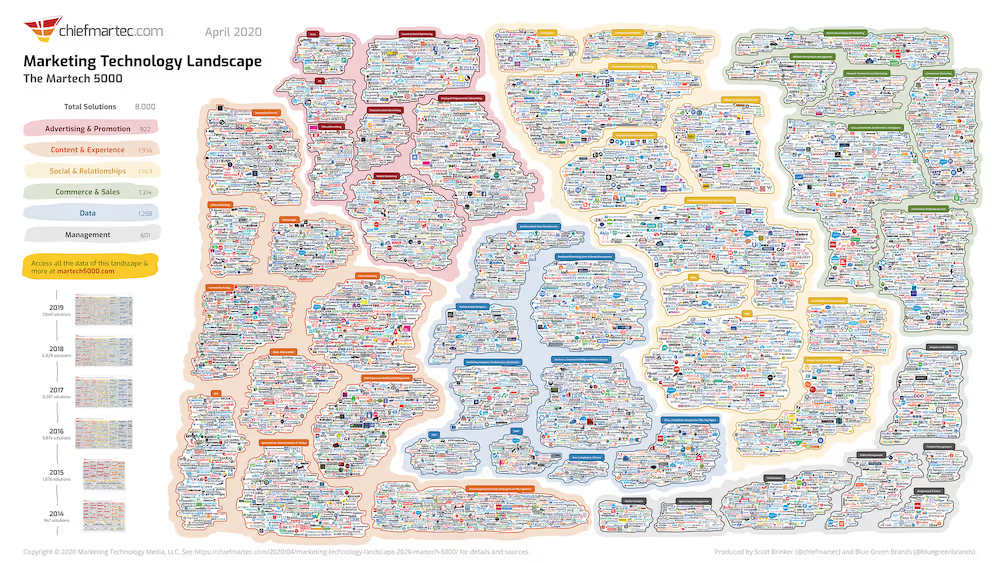
Your goal should be to find a solution to break those silos by creating a single view of your customers.
2. Raw data you can apply your own metrics to
Most digital teams start with Google Analytics to get an overview of what their customers are doing online. But such tools apply their own definitions e.g. what’s classed as a “conversion”, “churn” or “session”. But this might not align with how your business defines these metrics.
Ideally you’ll want a solution that allows you to define your own metrics, models, KPIs, and goals to the raw data. Meaning you can change and apply different logic while maintaining the original data.
3. Unsampled and unaggregated data
Many data tools aggregate or sample your data. E.g tools such as Google Analytics impose sampling onto your data when you create ad-hoc queries that reach their thresholds for sampling, which are:
- For Analytics Standard accounts: 500k sessions at the property level for the date range you are using.
- Analytics 360 account (paid): 100M sessions at the view level for the date range you are using.
Google classes an ad-hoc query as;
“If you modify a default report in some way—for example, by applying a segment, filter, or secondary dimension—or if you create a custom report with a combination of dimensions and metrics that don’t exist in a default report.”
So effectively as soon as you try to analyze the data in anything but the default way, Google gives you a sampled set of data to work with. There are three main issues that can arise from using sampled data;
- If the sample size is small your findings might not be representative of your whole data set.
- The more traffic you have the more inaccurate your sample may become.
- Sampling can lead to inaccuracies such as GA reporting different revenue to your actual financial/sales data.
You’ll want to find a solution that doesn’t automatically apply sampling unless you decide to do so.
4. Choose your attribution model
Yes, most tools (Google Analytics, Facebook Ads, etc.) have their own reports but they all use models that best fit their (not your) interests. Also, attribution in most data tools is optimized for a single action (e.g. lead submit or purchase) but what if you want to measure different channels on metrics like retention and Customer Lifetime Value (CLTV)?
Depending on your business, you’ll likely want to go beyond the basic first click or last non-direct click models and get familiar with models like Markov Chains or machine learning-based attribution. Both of these allow you to apply different attribution models to your data.
5. Create useful dashboards and reports
Because you can define your own metrics and definitions, set your attribution model, and have unsampled data, it means that dashboards and reports become a lot more useful for those within your business. Not to mention you’ll have data from all of your tools/sources so you can now put the data together to get information on how users progress through different customer journeys.
Building reports is probably the best way to get to know your data – both how it’s structured and what kinds of insights it might hold.
As we’re talking about customer data, you’re most likely interested in metrics like CLTV and retention rates. You might already have some information about what tends to move those metrics in either direction. Now you can start validating your hypotheses by analyzing which are the best performing segments, what triggers certain behaviors, etc.
There are numerous ways you can leverage your customer data but here are a few ideas to get you started.
- Marketing automation - target people with campaigns based on their behavior. Spend more on targeting people that are similar to your highest value segments/customers
- Rework your marketing budget - now that you have a much better overview of the attribution, make the necessary adjustments to your marketing spend to drive higher profits.
- A/B testing - no better way to validate your ideas/hypotheses than running an A/B experiment.
- Machine learning - you have tons of valuable data. No human being can work through it all, leverage machine learning to build things like better product recommendations, targeted messages, and other on and off-site personalizations.
So now you know what you can achieve, it’s time to work out which is the best solution - CDP or data warehouse?
What’s the difference between a Customer Data Platform (CDP) and Data warehouse?
There are a ton of tools and technologies out there with overlapping features, making it difficult to discern between them. Some of the popular names you might hear are Customer Data Platforms (CDPs), Data Management Platforms (DMPs), Data Warehouses, and Customer Relationship Management (CRM) systems.
Because of a general lack of commonly accepted definitions and blurring of functionally among vendors, we often see different business functions choosing different tools and approaches which can lead to a hodgepodge of tech stack. Not ideal.
Let’s discuss the differences between a Data Warehouse and a Customer Data Platform (CDP) to add some clarity about your options.
What is a customer data platform?
According to the Customer Data Platform (CDP) Institute, a CDP is a;
“Packaged software that creates a persistent, unified customer database that is accessible to other systems”.
Popular CDPs include Hull, Oracle, SAP, Adobe, Salesforce, Teradata Tealium, Acquia CDP, BlueConic, and Lytics.
The CDP industry is still relatively new and recent acquisitions of CDPs (e.g. Twilio acquired Segment for $3.2 billion), has only spurred other data software companies to try to muscle in on offering CDP functionality.
What is a data warehouse?
“A data warehouse (often referred to as an Enterprise Data Warehouse, or EDW) is a central repository in which large amounts of business information, from multiple departments and data sources, are integrated.
Data warehouses contain data from sales, marketing, purchasing, finance, (really any and all business functions.) The data, whether about employees, customers, support tickets, infrastructure, finance, etc, is generally held in one big pool, a single, reliable source of information, that any department or stakeholder can potentially access for reporting and analysis purposes.” - Source
Popular data warehouses include Google BigQuery, Snowflake, Amazon Redshift, or Microsoft Azure Synapse.
What’s the difference?
While both tend to use the same underlying technologies, data warehouses are generally used as a core piece of infrastructure for business intelligence, reporting, and data analysis. Whereas CDPs are generally chosen as a software to assist in marketing activities.
Despite the similarities in technology, their features differ a lot. This handy table helps identify the similarities and differences between the two.
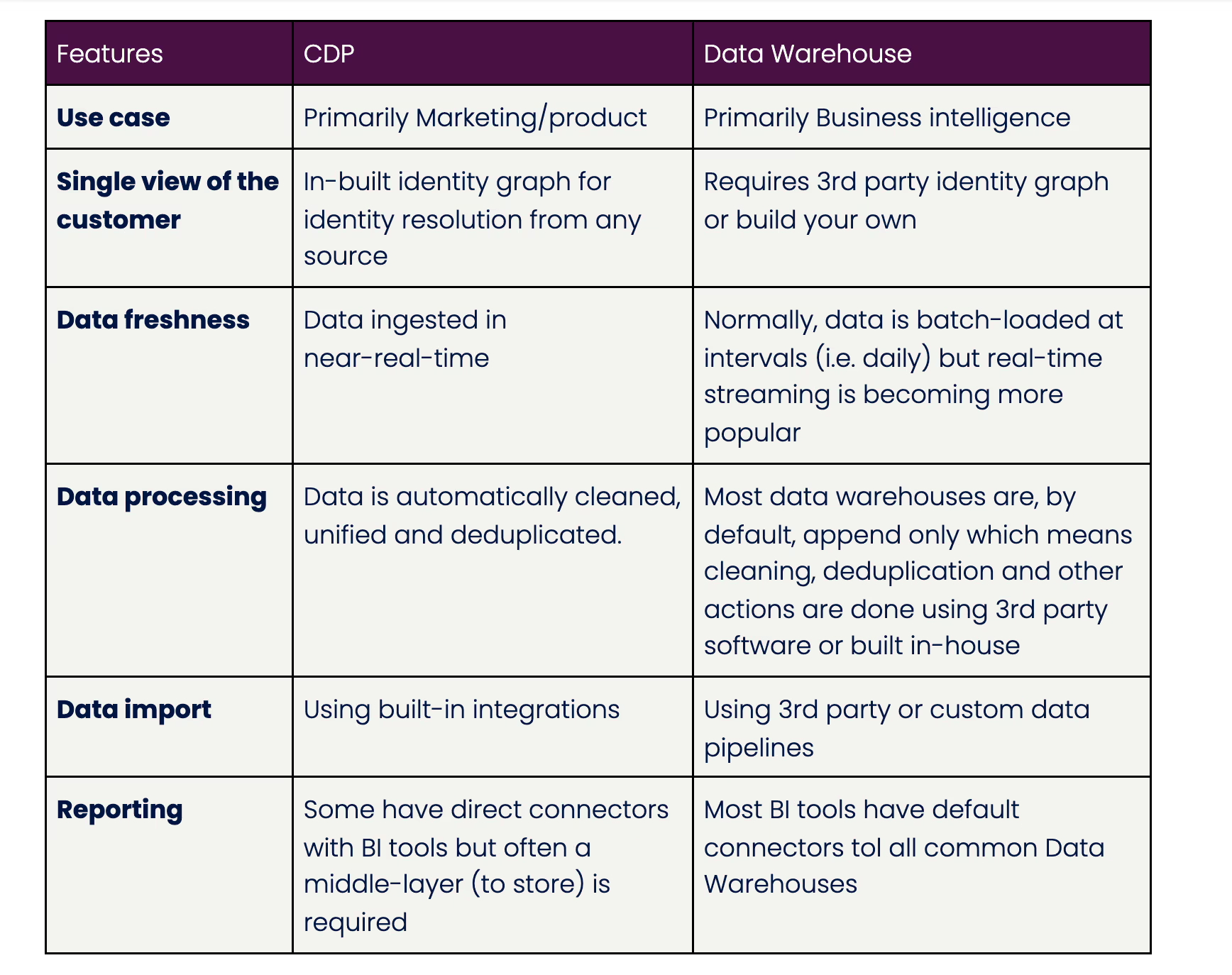
CDPs in my opinion should really be viewed as software that sits on top of your existing data infrastructure to enable marketing/product teams to answer most of their customer and analytics needs.
If you have smaller data sets and more basic data needs then a CDP is likely the easiest and quickest way to get real time data, in a way that’s easy to view and action for marketing/product teams. But there are a number of issues CDP users run into (more on that below) so it is not necessarily the silver bullet to all your data problems.
The data warehouse route is more advanced. It requires technical expertise to set up and manage, but with that you gain more control over how you can use your data, and the models you can apply to it. If your business has larger data sets, and wants to explore advanced or custom ways to use the data, this is a better route. And given the functionality available with modern cloud data warehouses, I’d tend to plump for this option rather than relying on the offering from CDP software.
Let’s look at the pros and cons of each option.
Pros and Cons of CDPs/Data Warehouses
Accessing the data
A customer data platform is a single tool, a piece of software that’s built for the purpose of aggregating data from multiple sources to create unified profiles of your customers. This means that getting started is often as simple as connecting/authorizing your data sources through out-of-the-box integrations and waiting for the CDP to collect some data. This is, of course, if the CDP you chose has an integration for all of your data sources.
If you’re considering a CDP your first point of call should be to find one that has all the integrations for the data sources you need today and in the near future (they usually list their integrations on their website.) If you can find a CDP that meets at least most of your data source requirements and has an API to pull data from other sources using a custom pipeline then that’s half of the battle.
Most CDPs have a user interface where you can log in and start exploring your data. In most cases, you can even build reports and data visualizations right within the same user interface.
With a customer data platform, accessing data on a basic level is really easy. That being said, you’ll likely hit limitations of the UI sooner or later, and then your next step will most likely be a business intelligence (BI) tool.
When it comes to accessing the data stored in a data warehouse, though, it’s a quite different story. You are either going to need to use a query language like SQL or a third-party tool like Looker or Tableau to access your data.
Again, complexity is the price your pay for the (almost unlimited) flexibility when working with your data. These days, there are integrations between all major CDPs, data warehouses, and BI tools. There may be some technical work to get it up and running (defining the schemas, data models, etc.) but once it’s done then it’s no more difficult than using a spreadsheet.
Costs
According to Gartner, the cost to purchase a CDP can range from $100,000 to $300,000 annually, usually depending on the amount of data you have. However I believe for smaller businesses you could likely start at around $10k for a CDP. Saying that you are still likely to pay 10x less for a data warehouse.
Data warehouse costs can be harder to pin down. Costs depend on a number of factors such as;
- Whether you build the warehouse from scratch or use a DWaaS (Data Warehouse As A Service)
- Where your warehouse is (on-prem or cloud)
- The amount of data you have to store, number of users or queries you run.
- There are also staff/contractor costs for set up and maintenance. Although newer DWaaS offerings such as Panoply are driving down the price as well as the amount of engineering support you need.
- You also need to factor in additional tool costs such as ETL tools/data pipelines, BI tools, visualization tools etc.
Security
With a data warehouse the responsibility for setting up the security practices falls to you. But with a CDP some of the security protocols will already be created for you to follow and use.
But at the same time, with a CDP you don’t have control over their data security practices and so if a data breach happens the management of that is out of your control.
Limitations
With a CDP you are generally limited by their features, models and capabilities (similar to some of the problems you are trying to overcome by using such approaches in the first place.) E.g they might offer pre-defined “personas'' but you can’t go beyond that to customise them based on your own models, say based on sessionized data or points in a customer journey.
The same limitations are there too when it comes to the analytics side of CDPs. Most CDPs focus on creating segments of customers based on behavior. But generally the analytical capabilities and models are pretty simplistic (which is why they can offer intuitive user interfaces for non-technical teams out of the box). If you want to apply machine learning or artificial intelligence to your data you’ll need to use another tool to do so. Meanwhile Snowflake and Google are creating machine learning algorithms that can be executed within your data warehouse.
Some data warehouses work better with different kinds of data than others (SQL vs noSQL, append-only, transactional, relational, etc.) but generally, they don’t guide or limit you much.
And because of the nature of a data warehouse (more of a multi-purpose technology that’s designed to store data. Lots of data) there are no real limitations in terms of what’s possible.
On the flip side this means you are responsible for defining the schemas, tables, relations between data points and almost everything else about your data. This flexibility comes at a price; more work to get up and running. However, there are ready-to-use data pipelines for almost all common data sources and data warehouse providers.
How to decide?
While there is no right or wrong here, my general rule of thumb is that a CDP is likely the better option if;
- You need a way to view and use data without much technical/analytical resources
- You use common/popular non-custom tools–so the majority of the integrations are already there.
- You don’t want to apply advanced models or analysis techniques.
- It’s mainly marketing use cases for the data.
On the other hand, if you have multiple teams relying on this data outside of your marketing team and you want ownership and flexibility to run advanced analysis, personalization, etc. a data warehouse is a better choice.
The reality is that with new tools and technologies coming onto the market every day, you can effectively achieve the same outcome with either option (just in different ways/costs). For example; you can integrate your CDP with tools that allow you to do more advanced analysis. While you can use data layer tools and pipelines to cut down on building things from scratch for your data warehouse. E.g. RudderStack is a data pipeline that builds your identity graph in your warehouse and connects with other data tools.